Faster, easier geographic data cleaning with LinkSight
October 2, 2018
Imagine that you work in a disaster response organization. A destructive typhoon has hit several Philippine provinces across Luzon. It’s your job to consolidate all the damage reports submitted by a network of field personnel. Your boss asks you to summarize and map the reported damages for hundreds of affected barangays. Easy peasy, right?
Not quite. Your data is a hot mess. People have used different spellings to refer to the same location, such as “San Isidro”, “St. Isidro”, and “Santo Isidro.” People have also used different abbreviation conventions, such as “Brgy” and “Bgy.” You painstakingly check and correct each entry, spending hours on a task that you expected to take just a few minutes.

This is an all too familiar problem for organizations working with Philippine location data. Misspellings, abbreviations, inconsistent styles and conventions make it tricky to identify the same place across different records. We regularly encounter this issue at Thinking Machines, especially when we’re trying to join multiple data sources to do geospatial analysis and mapping.
A faster, easier way to clean geographical names
So we thought, why not semi-automate this process? That’s why we’ve built LinkSight, an open-source web-app that will clean up messy location datasets, quickly and easily. No coding required.
Linksight uses a fuzzy matching algorithm to take a list of inconsistently spelled location names and find their closest matches in the Philippine Standard Geographic Code or PSGC. The PSGC is an authoritative list of the official names of every barangay, municipality, city, province, and region in the Philippines. Maintained by the Philippine Statistics Authority, the PSGC also uses 9-digit ID numbers to uniquely identify each administrative territory.
Using LinkSight is simple. You just upload a CSV file with location names and run the matching algorithm. If LinkSight isn’t 100% sure about a match, it will ask you to choose the correct one from the top 5 closest matches.
Once you’ve confirmed all the correct matches, you can export a new CSV file that will have the standardized location names and PSG codes as additional columns.

How is this useful? The PSG code added by LinkSight can be used as a unique identifier to differentiate similarly named places and as a primary key to connect several datasets. Following standards like the PSGC also make it possible to enrich your data by linking it with external datasets. For example, we recently published a map that shows the natural hazards to which each of the Philippines' 42,000+ barangays are exposed. You can join your own barangay-level data with maps like these by using the PSG as a common link.
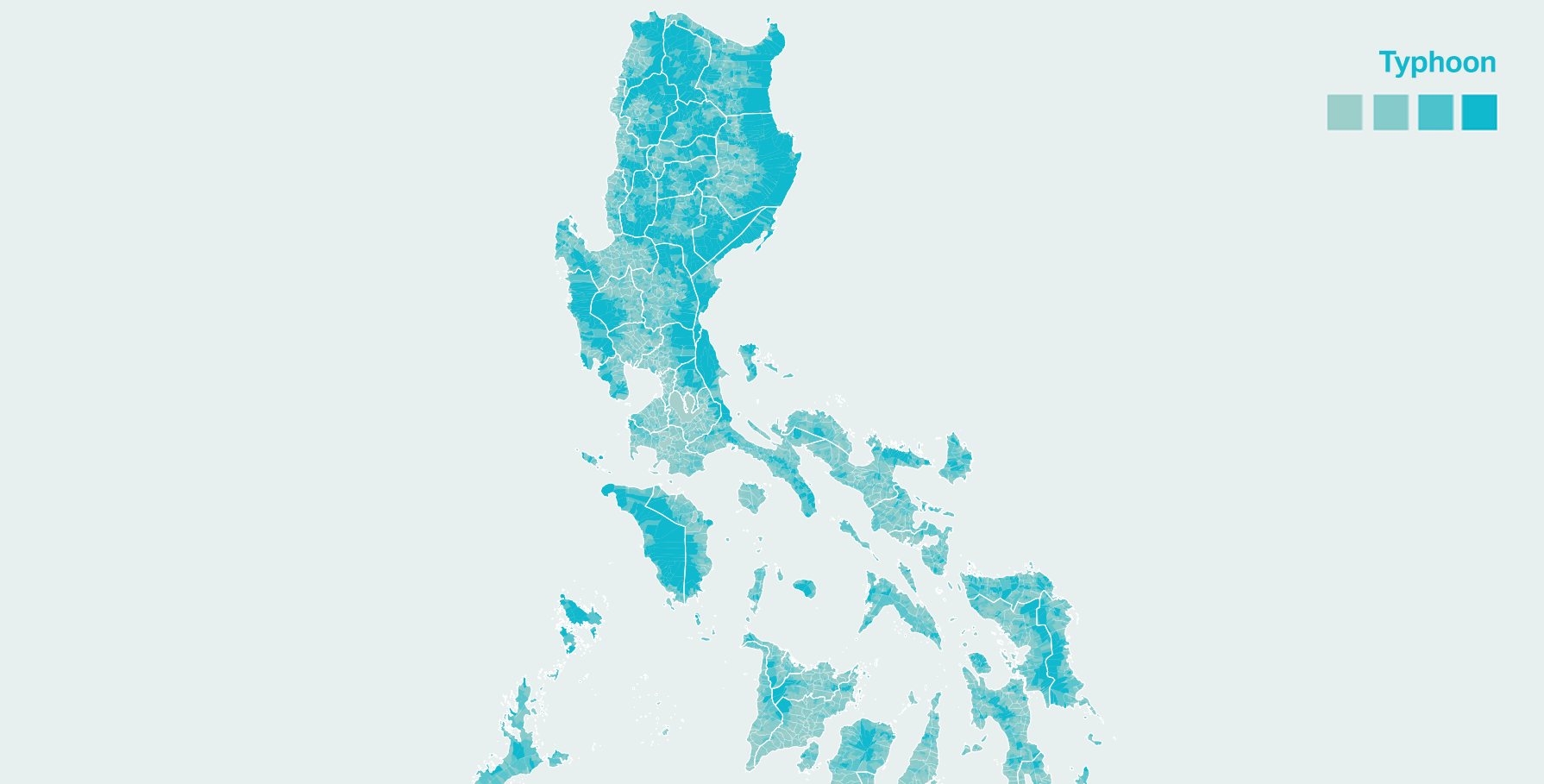
With cleaning of location data made simpler, organizations can focus on conducting data analysis, finding insights, making data-driven decisions, and creating impact.
Help test our prototype
We’ve released an alpha prototype of the product for a limited group of volunteer testers. Over the next few months we’ll be conducting user testing sessions to collect feedback on how to improve the tool even further.
If you’re an organization or individual interested in using the tool, email us at [email protected] or sign up for access here. We’re interested in hearing your thoughts, ideas, and suggestions for how this tool can be improved.
We’re also testing different record matching algorithms that our engineers developed to find which one has best speed and accuracy performance. If you’re a developer interested in cracking this puzzle with us, you can contribute to our repository on Github.
We’re building LinkSight through the support of the UNICEF Innovation Fund, which finances early stage, open-source technology solutions for the development community.
Watch this space for more updates.